Electrical Engineering and Computer Science (EECS)
Among the leading departments of its kind in the nation, EECS is creating the technology that puts the “smart” into electronics. Our excellence and impact comes through in the work of our two divisions.
EECS at Michigan
Established. Respected. Making a world of difference. EECS undergraduate and graduate degree programs are considered among the best in the country. Our research activities, which range from the nano- to the systems level, are supported by more than $75M in funding annually — a clear indication of the strength of our programs and our award-winning faculty. With this combination of great resources and talent, EECS at Michigan is transforming and improving a wide range of fields that touch all of our lives.
Tools for “more humane coding”
Prof. Cyrus Omar and PhD student David Moon describe their work to design more intuitive, interactive, and efficient coding environments that can help novices and professionals alike focus on the bigger picture without getting bogged down in bug fixing.
Snail extinction mystery solved using miniature solar sensors
The World’s Smallest Computer, developed by Prof. David Blaauw, helped yield new insights into the survival of a native snail important to Tahitian culture and ecology and to biologists studying evolution, while proving the viability of similar studies of very small animals including insects.
Events
News
James Juett voted 2024 HKN Professor of the Year
Dr. Juett is receiving this honor for the second time.
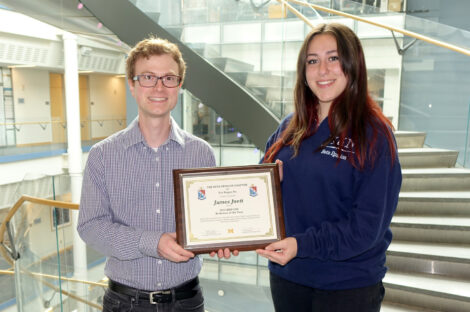
Poster session showcases student-developed GenAI software systems
Prof. Mosharaf Chowdhury’s Systems for GenAI course closed with a poster session highlighting student projects.
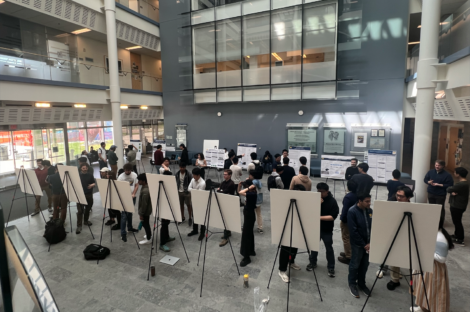
Stéphane Lafortune voted 2024 HKN Professor of the Year
Prof. Lafortune is receiving this honor for the second time. Last year, he taught the introductory course in signals and systems, and a new introductory course focused on the challenges…
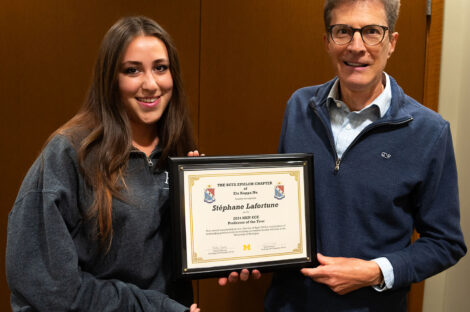
EECS By the Numbers
Members
Research Expenditures
University
Students, F 2021