Michigan Systems Laboratory
Faculty at the University of Michigan Computer Science & Engineering Division whose work spans distributed systems, operating systems, security, cyber physical systems, networking, databases, and software engineering.
News
Poster session showcases student-developed GenAI software systems
Prof. Mosharaf Chowdhury’s Systems for GenAI course closed with a poster session highlighting student projects.
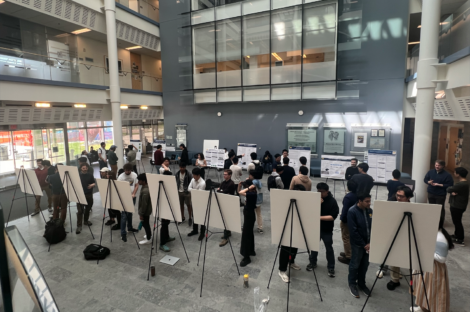
CSE researchers win Best Paper Award at ACM MMSys 2024
The authors were recognized for the excellence of their research on neural-enhanced video streaming.
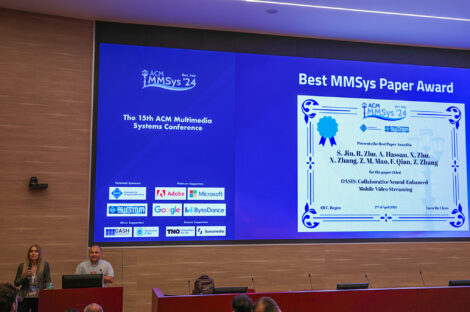
Roya Ensafi receives Willie Hobbs Moore Achievement Award
The award recognizes Ensafi’s outstanding achievements and contributions to promoting equity in STEM.
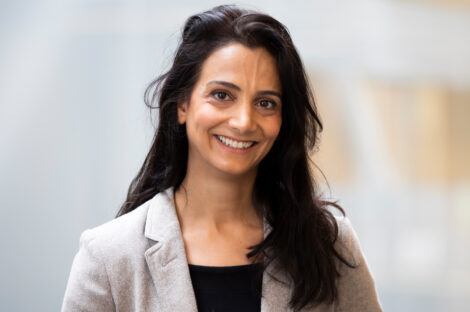
Tools for “more humane coding
Assistant professor Cyrus Omar and PhD student David Moon describe their work to design more intuitive, interactive, and efficient coding environments that can help novices and professionals alike focus on the bigger picture without getting bogged down in bugfixing.
Making complex software more reliable
Assistant professor Manos Kapritsos, a researcher in CSE’s Systems Lab, studies how formal verification can be used to ensure even the most complex, distributed systems can be guaranteed correct without testing.